Use cases
Monitoring and improving data quality
quality
Sensor data (IoT), financial transactions, plant information, anomaly detection
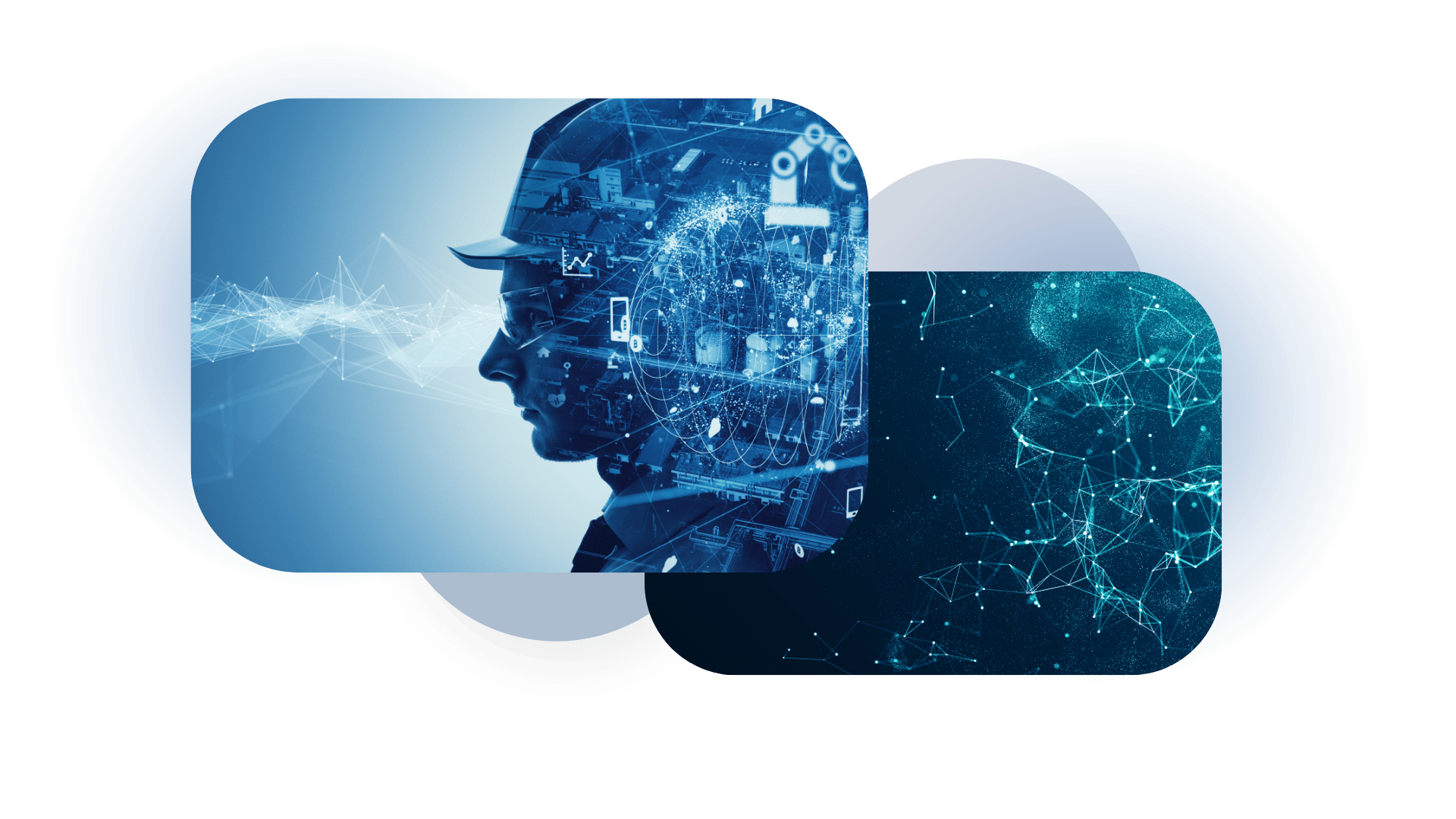
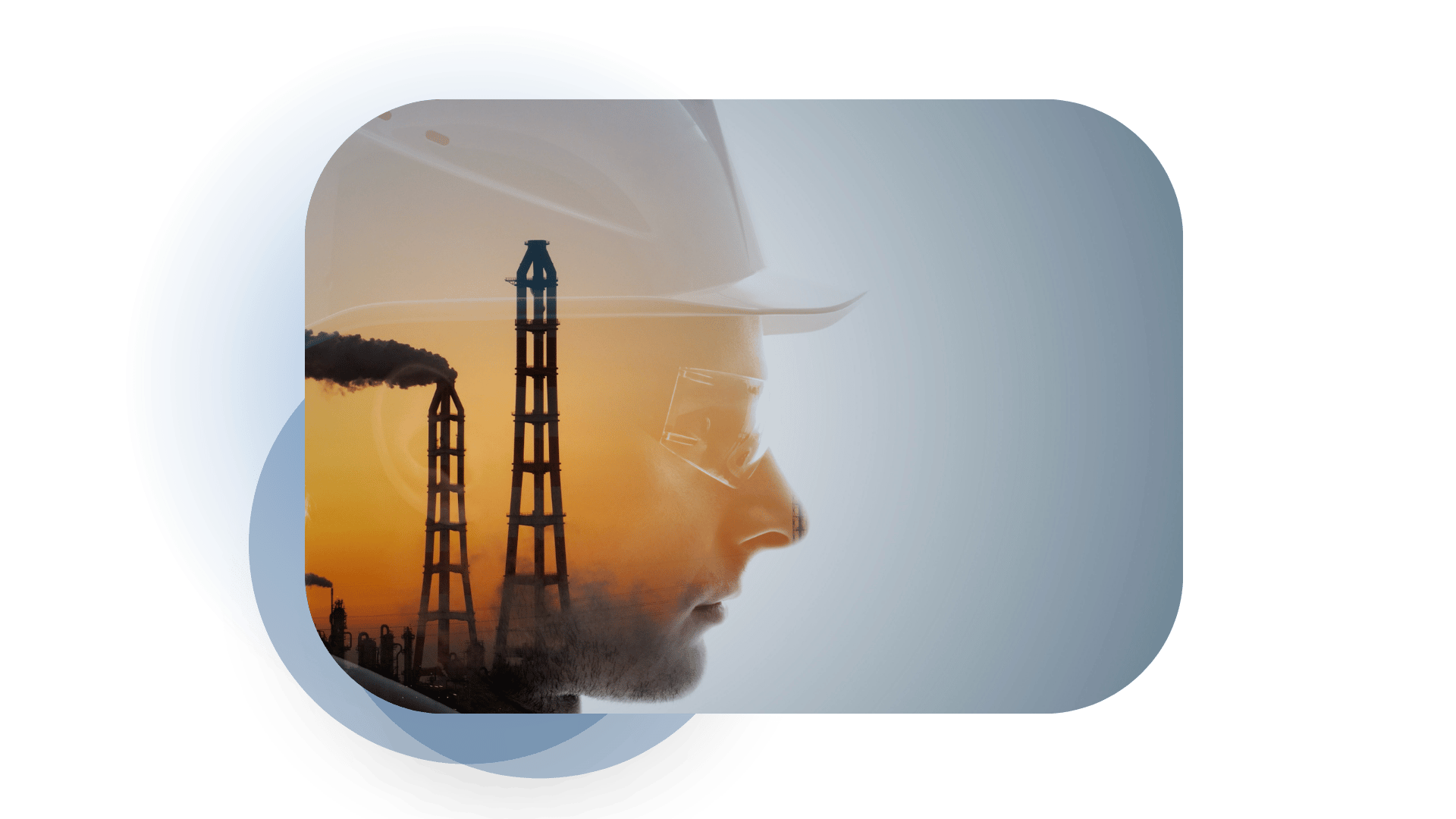
The requirement
Our customer, an industrial group with hundreds of subsidiaries worldwide, wanted to control and improve the quality of PI data (PI = Plant Information: data emitted by sensors installed on production sites).
The objectives were multiple:
- To have PI nomenclatures (Assets, Attributes, Tags) with clear naming rules, which are free of duplicates to enable better tag reuse and cross-site analysis.
- Set up a high-performance monitoring system for PI Tags (= time series): real-time detection of missing or aberrant data, identification of faulty sensors, etc.
- Supply teams of Data Scientists with reliable data, an essential prerequisite for building coherent, high-performance predictive models (forecasting, predictive maintenance, etc.).
Proposed solution
Harmonization of sensor nomenclature:
Tale of Data automatically matches texts (name, description, etc.) with spelling differences using advanced fuzzy matching algorithms: phonetics (English/French), consonant (or vowel) frequency, word fragmentation (N-Gram), or automatic word weighting: the least discriminating words are given a low weight.
Monitoring sensor data using Tale of Data's time series analysis algorithms:
- Determination, by sensor type, of appropriate alert thresholds for measured values (temperature, pressure, etc.): these thresholds were obtained by running an automatic analysis over several years of historical data.
- Determination, by sensor type, of appropriate alert thresholds for time gaps between two measurements: these thresholds were obtained by running an automatic analysis over several years of history.
- Automatic alerts when thresholds are exceeded or data is missing.
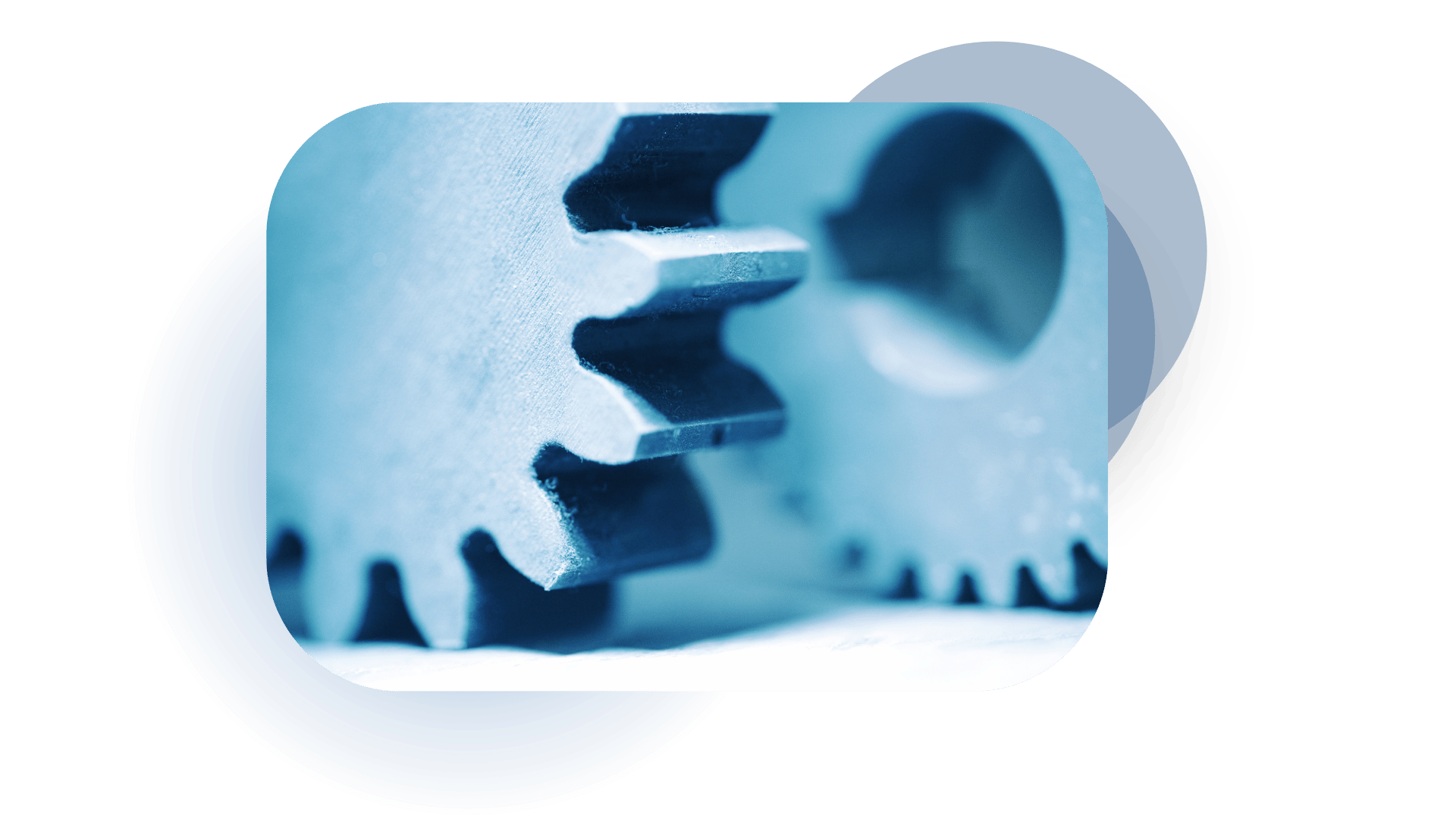
.png)
Gains achieved
The harmonization of labels and deduplication has enabled the creation of a shared PI metadata repository: Assets, Attributes, Tags.
This shared PI metadata repository, with clear naming rules, has opened up numerous possibilities:
- Consistent representation of the system: same set of attributes for elements representing the same type of equipment, with standardized names, descriptions and units of measurement
- Facilitation of "multi-point" analyses: standardized metadata make it possible to aggregate or compare time series, whether for monitoring, reporting or predictive analysis (Machine Learning ).
In just a few weeks, time-series analysis enabled us to put into production a fully automated monitoring system, continuously analyzing data from tens of thousands of sensors.
Alerts on very specific conditions have been set up (sensors emitting erroneous values or showing anomalies in the time intervals between two measurements). These alerts can be reconfigured at any time by business users, without writing any code.
Product Benefits
Integrate
Seamless integration of AI and No-Code technology for effective data refinement.
Collaboration
Shareable
Visualize
Powerful
Quality
Achieve superior data quality faster and at a lower cost, while demonstrating the tangible value of investing in data excellence.