What is Data Quality? Everything you need to know to master data quality in the workplace
What is Data Quality? Everything you need to know to master data quality in the workplace
In a world where data drives every strategic and operational decision, data quality is essential. Imagine a brilliant artificial intelligence algorithm, designed to predict trends with frightening accuracy, but fed with erroneous or obsolete data. The result? Biased forecasts, missed opportunities, lost credibility and lost sales.
Data Quality is an essential strategic issue for companies. From inventory management to predictive analysis, from regulations to performance management, data quality is the key to optimal operation and enhanced competitiveness.
In this article, we explore :
-
The definition and fundamentals of Data Quality.
-
The strategic and operational issues it raises.
-
Tools, criteria and approaches for guaranteeing impeccable quality.
-
Steps to initiate a structured approach in your organization.
Finally, we present "The Language of Data Quality", a glossary designed to harmonize practices and establish a common vocabulary within teams.
1. What is Data Quality?
Data Quality refers to an organization's ability to ensure that its data is reliable, consistent and fit for purpose. It is the foundation of all successful strategic and operational decisions.
Application example: maintenance management for an industrial park
Data Quality is not just a theoretical concept: it has concrete applications in the day-to-day running of companies. Take, for example, the management of a fleet of industrial machines, where every dimension of Data Quality plays a key role in performance and operational safety:
-
Accuracy: if a machine is declared "in good condition" but is in fact out of order, a critical intervention could be delayed, leading to costly interruptions.
-
Consistency: information on this machine must be identical in maintenance, inventory and production systems, to avoid errors such as unnecessary ordering of spare parts.
-
Completeness: fields such as "last overhaul date" or "parts replaced" must be filled in to correctly track maintenance history.
-
Freshness: if the status of a machine is not updated after a repair, this can lead to unnecessary interventions or poor performance monitoring.
-
Uniqueness: duplicate machine records can lead to errors, such as scheduling two identical maintenance operations.
-
Validity: serial numbers or part codes must comply with industry standards.
-
Integrity: a machine must always be linked to its location and its responsible operator.
-
Reasonableness: a machine with "0 hours" of use, even though it has been in production for a month, indicates an anomaly in data collection.
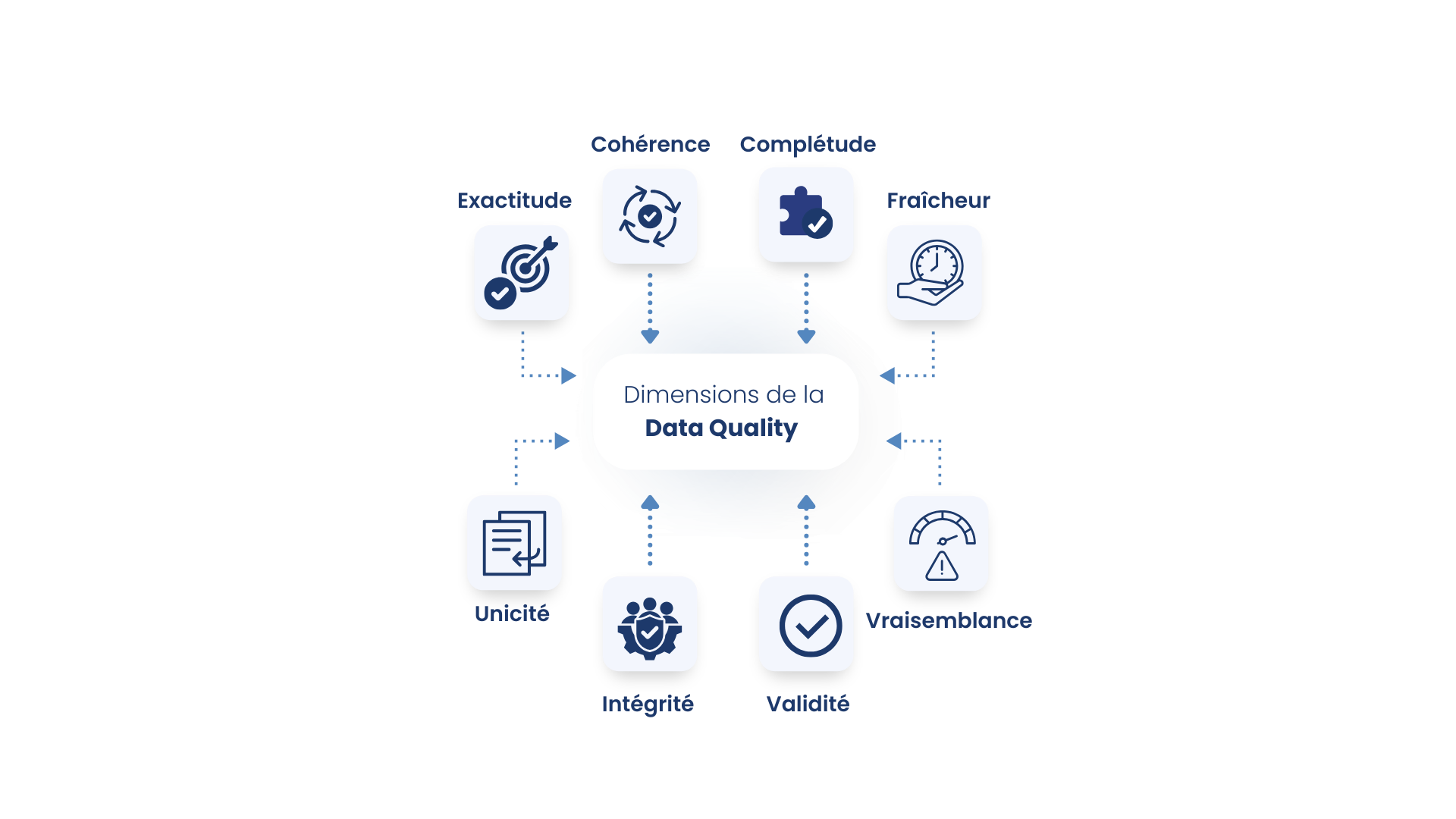
In this scenario, poor-quality data could not only disrupt operational efficiency, but also compromise employee safety, increase costs and reduce customer satisfaction.
To go further and master every dimension of Data Quality, download our exclusive glossary "The Language of Data Quality" free of charge . This practical guide, with over 70 key terms explained in simple terms, will help you transform your data into a real strategic lever and harmonize your business practices.
2. The challenges of Data Quality: why is it essential?
Strategy and competitiveness: taking full advantage of data
Operational performance: optimize and save
Regulatory compliance: protect and comply
Technical and cultural challenges: obstacles to be overcome
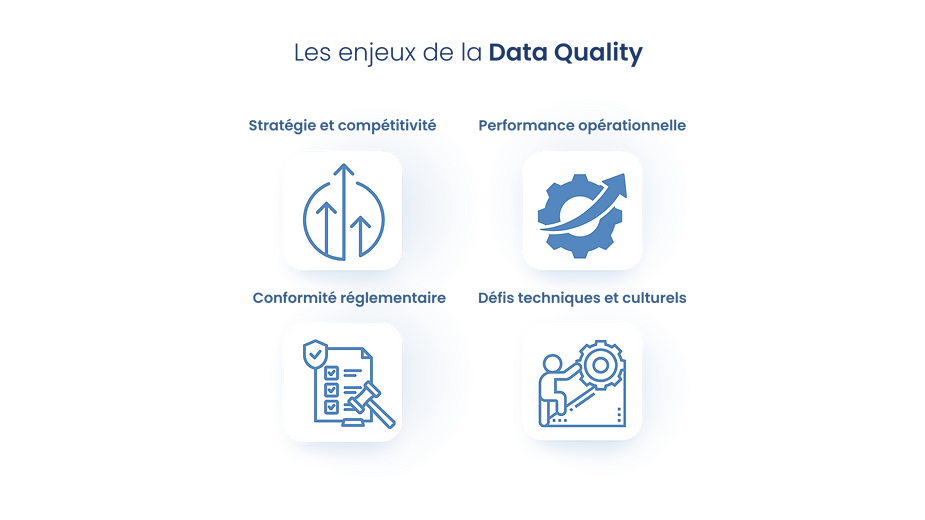
3. Tools and approaches for improving data quality
Improving data quality requires a structured approach that covers the entire data lifecycle. To meet today's challenges, companies need to adopt innovative solutions capable of transforming data management into a genuine strategic lever.
Comprehensive coverage with the 7 Segments of Data Quality
To meet the challenges of data quality, we recommend a 7-segment approach. These segments make it possible to manage all data transformation and enhancement processes, while integrating best practices:
-
Data Discovery: identify and explore your data to reveal its potential. Data profiling detects anomalies such as inconsistencies, duplicates and missing values.
-
Data Observability: continuously monitor your data flows to anticipate and resolve anomalies. Dashboards provide a real-time view of data quality.
-
Data Catalog: centralize and organize your data assets to simplify their use. This facilitates data cleansing, harmonizing formats and eliminating duplicates.
-
Data Quality: assess and improve critical data dimensions such as accuracy, completeness and consistency. Enriching data with reliable sources enhances its relevance.
-
Data Lineage: guaranteeing data traceability to ensure data integrity and compliance, a key asset in meeting regulatory and operational requirements.
-
Data Product: transform your data into usable products, bringing concrete value to business teams.
-
Data Stories: translate your data into clear, actionable insights through engaging visualizations, supporting decision-making.
Modern tools for every segment
Modern Data Quality tools go far beyond traditional functions. They integrate advanced features such as :
-
Intelligent matching: precise detection and matching of duplicates, even in the case of variations (e.g.: "L. Dupont" vs. "Laurent Dupont").
-
Window processing: data analysis over a specific period or context, such as daily performance monitoring.
-
Data observability: proactive monitoring to guarantee reliable data on an ongoing basis.
A solution designed for today's businesses
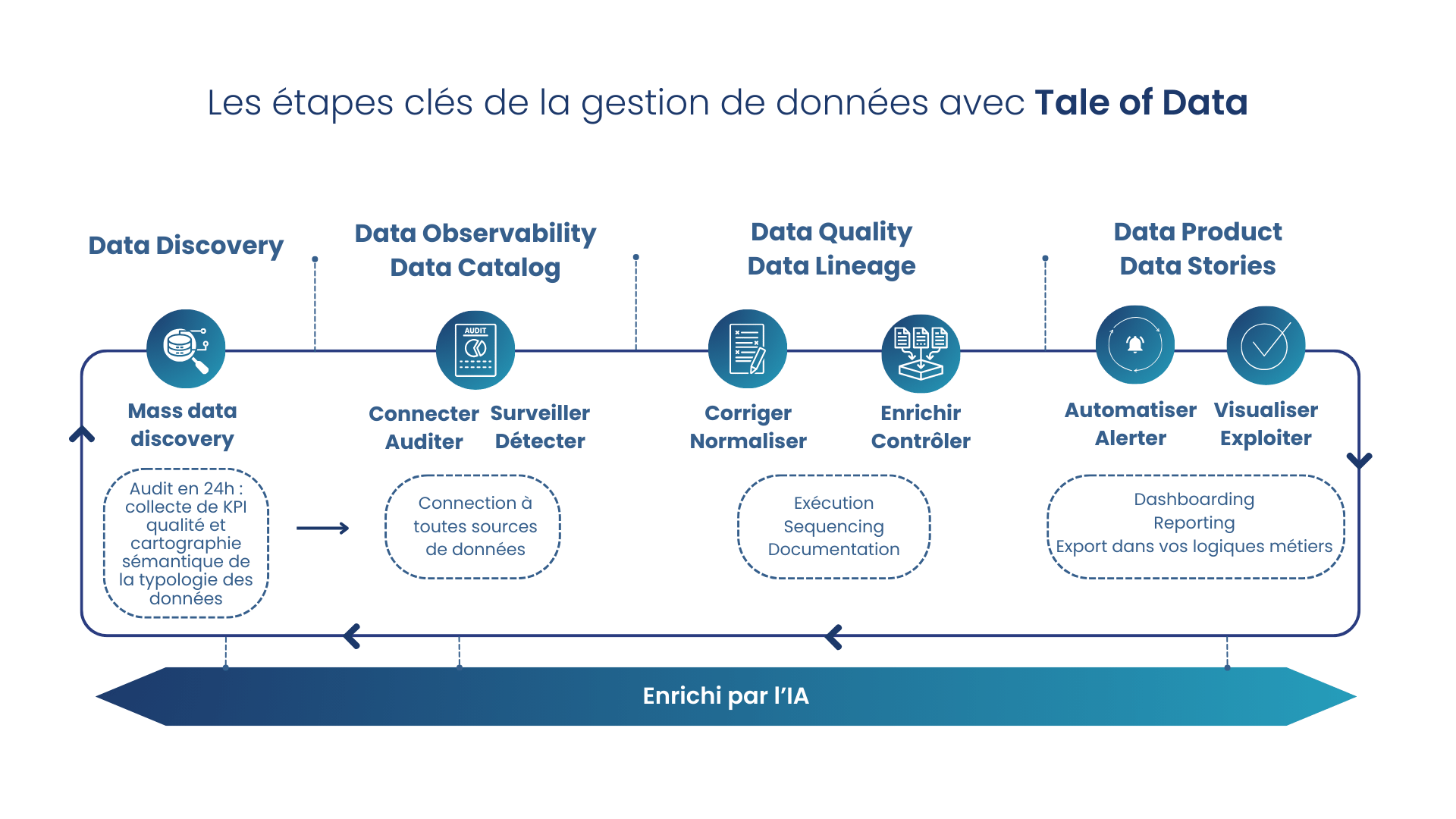
In this complex ecosystem, some platforms stand out for their innovative approach. With its AI integration and No-Code interface, Tale of Data simplifies data management by offering correction, enrichment and monitoring tools accessible to all. Whether you're a data scientist or a business manager, Tale of Data can help you transform your data into a genuine performance driver.
4. How to get started with Data Quality in your company?
1. Make a diagnosis
2. Set clear objectives
Once the diagnosis has been made, define precise, achievable objectives.
For example:
-
Reduce duplicate entries in your customer database.
-
Harmonize data between your CRM and ERP tools.
-
Ensure that 100% of critical information is in the right format.
Clear objectives will help you track your progress and stay aligned with your priorities.
3. Raise your teams' awareness
Data quality can't be solved with tools alone, it also requires collaboration between teams. To mobilize your staff:
-
Get everyone on the same wavelength with a common language, such as The Language of Data Quality.
-
Explain the benefits in concrete terms: more reliable analyses, fewer errors to correct, and time savings on a daily basis.
4. Equip yourself with the right tools
Automating repetitive and time-consuming tasks is essential. Choose tools capable of :
-
Detect and correct anomalies such as duplicates or inconsistent data.
-
Be easy to use for your teams, even without technical skills.
5. Monitor progress
Monitoring is essential to know whether your efforts are bearing fruit. Define simple indicators to measure :
-
Reduction of errors and duplicates.
-
Improved data consistency and freshness.
-
Operational and financial gains.
Dynamic dashboards enable you to visualize your progress in real time, and adjust your strategy if necessary.
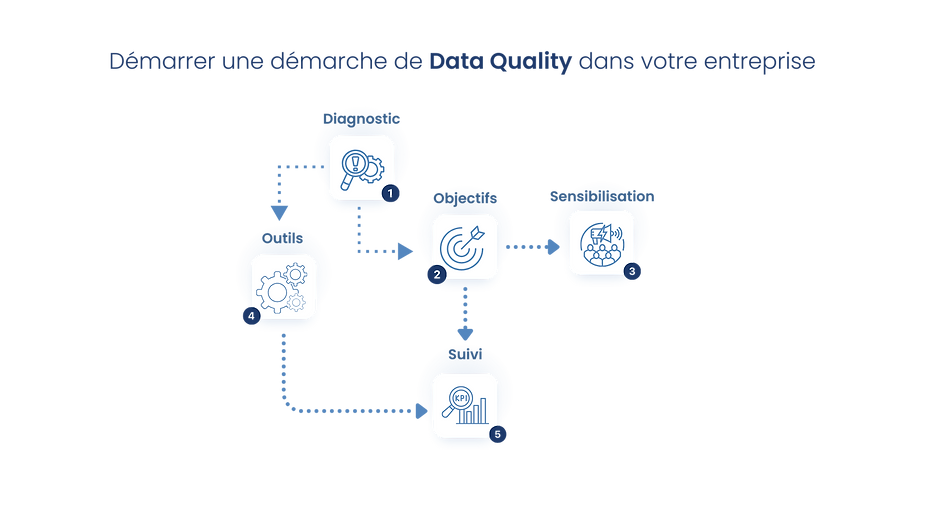
Adopting a structured approach and high-performance tools is essential to transforming your data into a strategic asset. Thanks to the combination of No-Code andartificial intelligence, solutions such as Tale of Data make data qualitymanagement accessible to all, with rapid learning curve and seamless collaboration between business and IT. With Tale of Data, every user - whether Data Scientist, Data Analyst or Citizen Data Expert - can manage data quality intuitively, quickly and efficiently, thus reinforcing the company's global strategy.
If you'd like to find out more about implementing a Data Quality strategy in practice, take a look at our practical guide to solutions and tools for successful data transformation:
Conclusion: Data Quality as a lever for transformation
💡 Not to be missed: an exclusive infographic summarizes all the key points covered in this article. Ideal for easily sharing best practices internally and raising awareness among your teams.👉 access the infographic here.

Data Quality goes beyond the merely technical: it embodies a strategic pillar for modern businesses. In a world where artificial intelligence and regulations are redefining standards, optimal data quality is essential to innovate, stay competitive, and ensure compliance.
To take things a step further and strengthen collaboration around data issues, "The Language of Data Quality" offersa common vocabulary to harmonize practices within your teams.
🌟 Why adopt this glossary?
-
Facilitate communication between business and IT thanks to a unified language.
-
Master key concepts such as Data Mesh, Window Processing or Data Freshness.
-
Maximize the impact of your data by aligning your teams around clear standards.
📥 Download "The Language of Data Quality" for free now and share it with your colleagues to build a strong data-driven culture:👉 download here.
By combining these resources, you'll be able to transform your data into a true strategic asset for your organization.
You May Also Like
These Related Stories
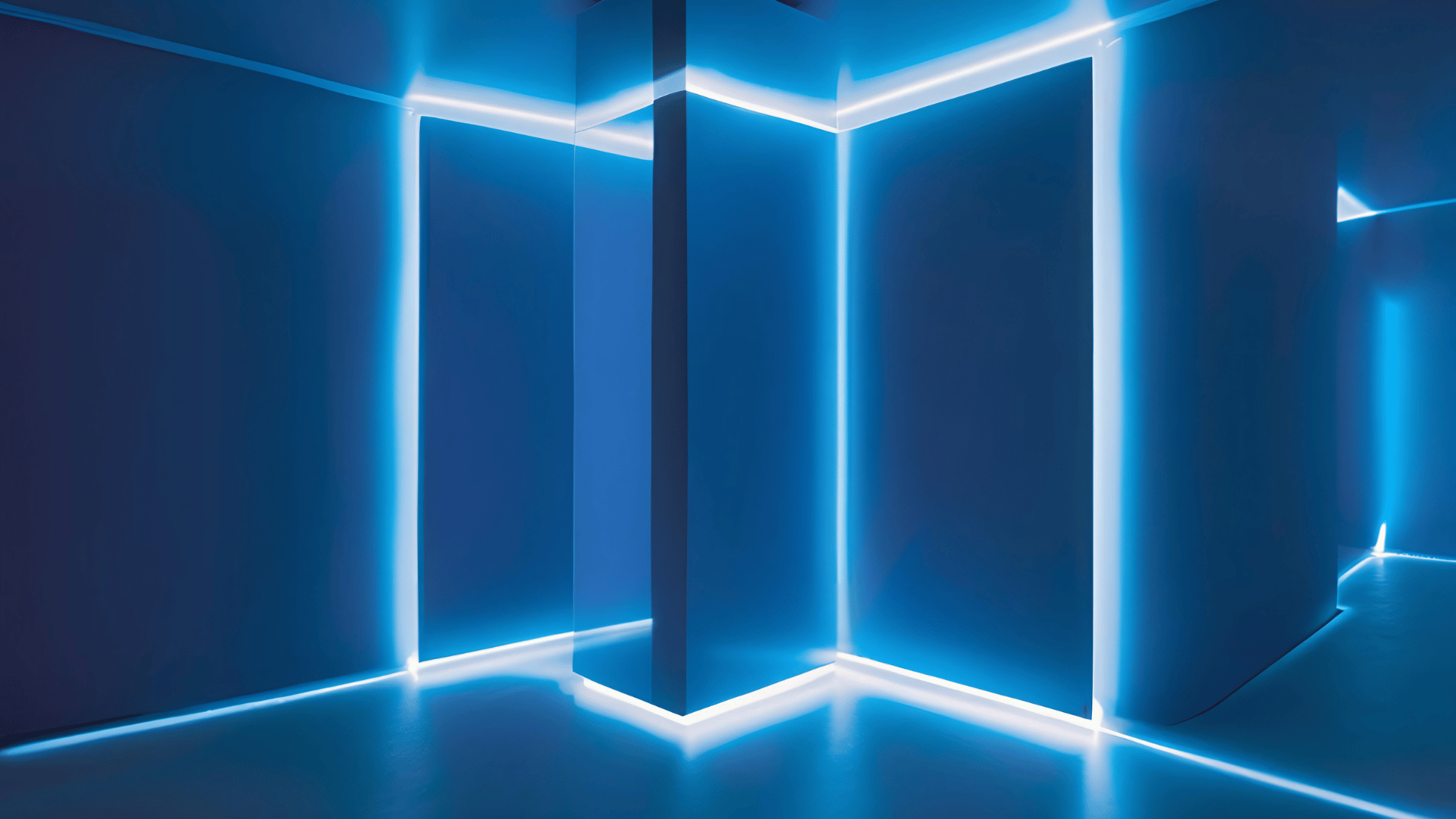
Data quality: how to implement a good data quality strategy?
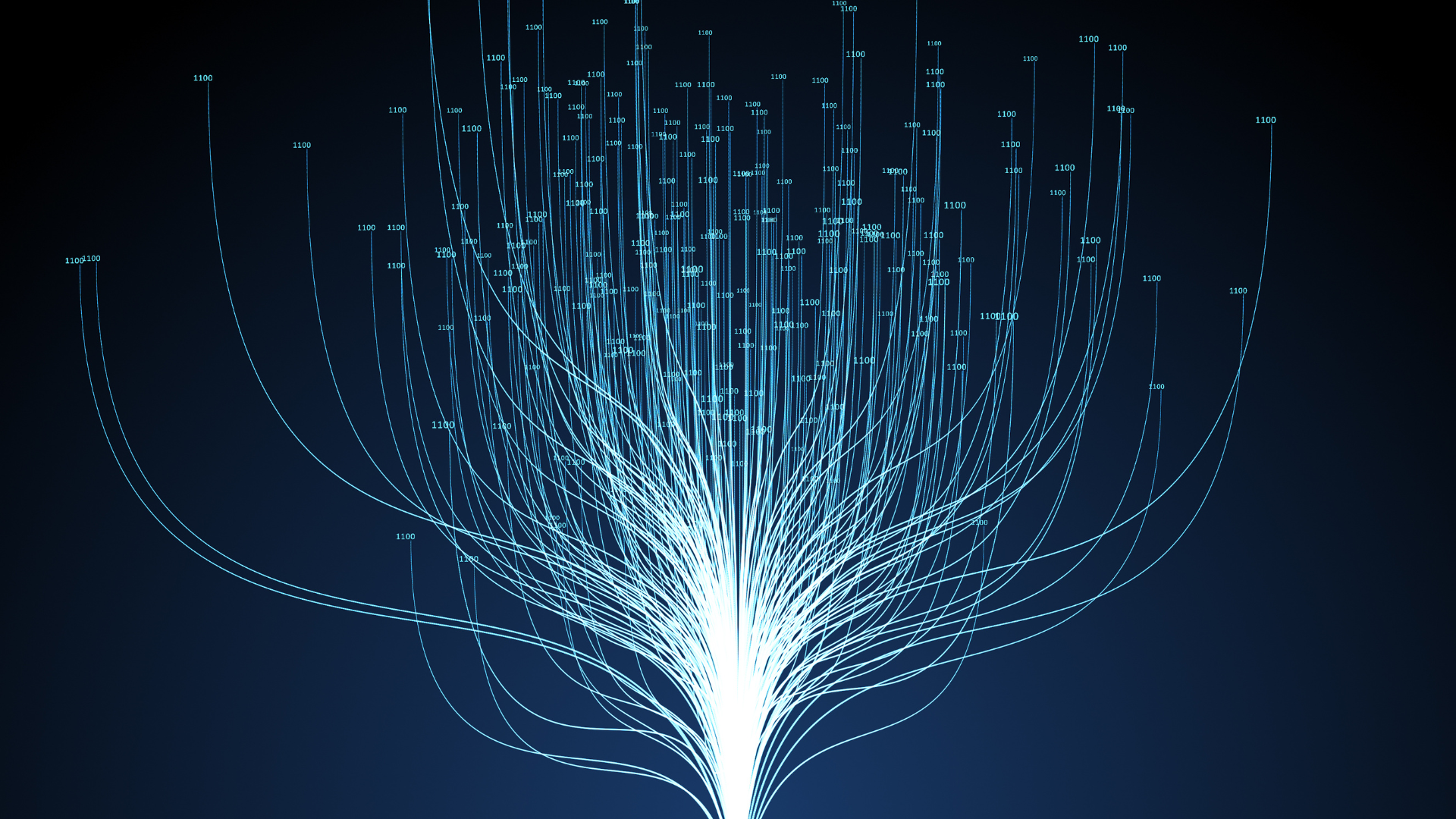
Generative AI to optimize data quality: Tale of Data's innovative approach
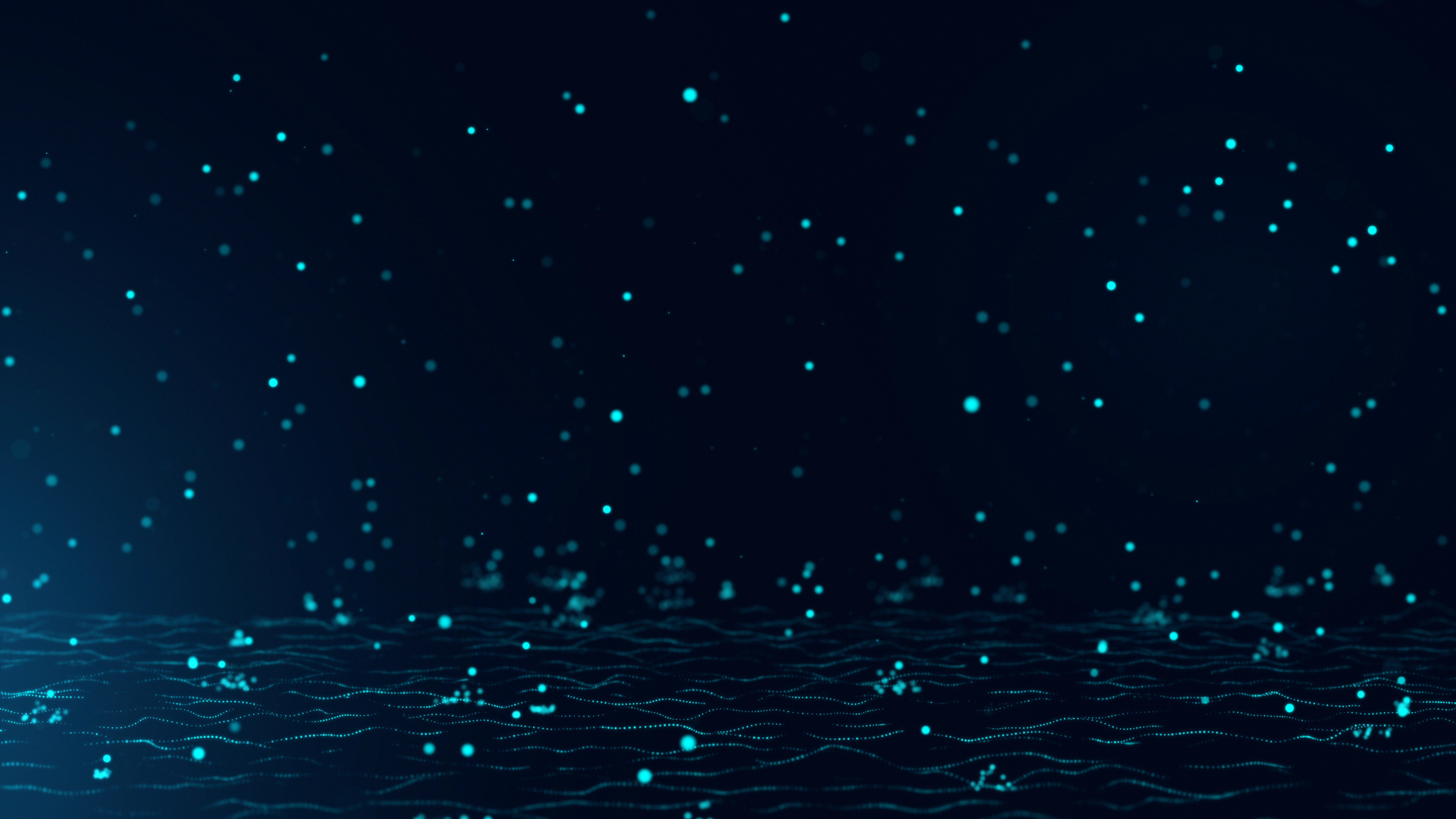